From the Source Code Perspective, Peering into the Operation Logic of LangChain
From the Source Code Perspective, Peering into the Operation Logic of LangChain
By interpreting the source code of Chain and AgentExecutor, let's understand how various modules are interconnected.
- Base Class of LangChain
- LCEL与Runnable
- Chain
- AgentExecutor
1. Base Class of LangChain
Python's abstract base class is ABC, and LangChain's base class is its subclass, Runnable。
The inheritance relationship of the base class is shown in the following diagram:
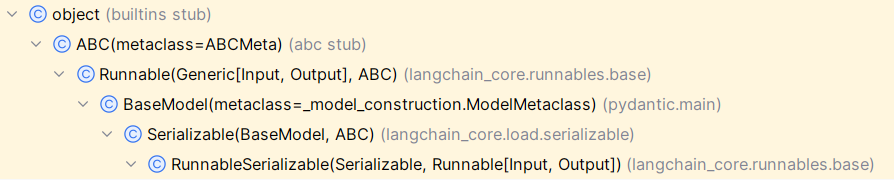
Subclasses of RunnableSerializable are as follows:
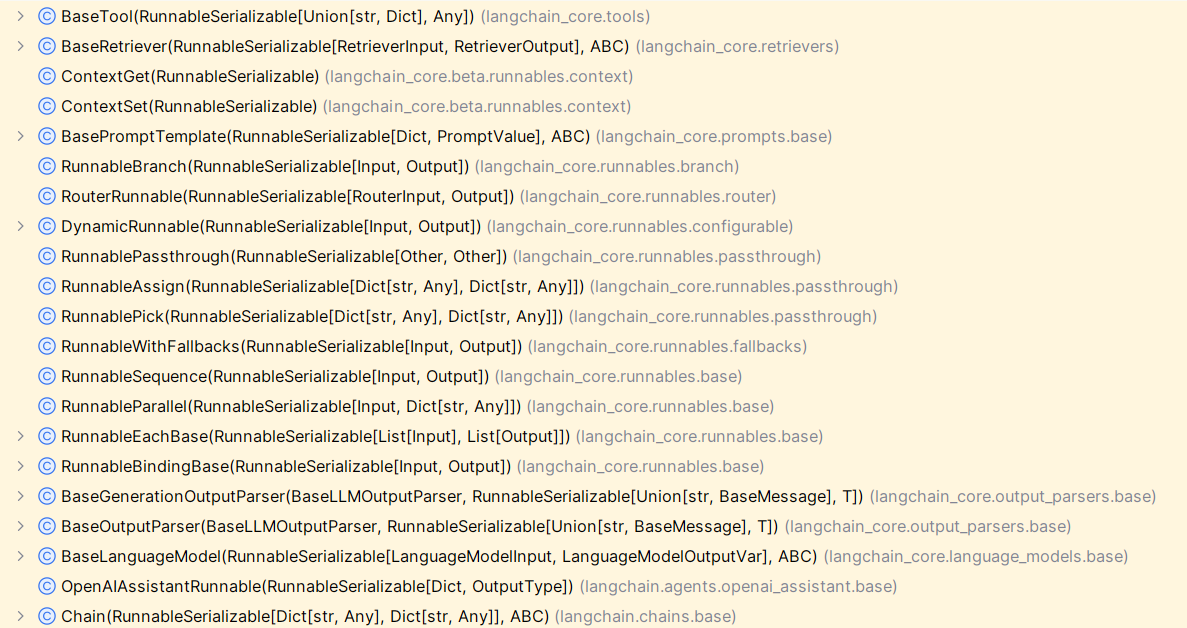
Extracting the important classes, we have the following inheritance relationship:
- ABC
- Runnable
- Chain
- AgentExecutor
- BasePromptTemplate
- BaseLanguageModel
- BaseOutputParser
- BaseRetriever
- BaseTool
- Chain
- Runnable
2. LCEL与Runnable
Intuitive understanding:
- Runnable is a component
- LCEL is a way to assemble components into a finished product
2.1. LCEL(LangChain Expression Language)
LCEL is a declarative way to easily combine Runnables into a Chain.
2.1.1. The Way to Compose Runnables
2.1.1.1. Serial(RunnableSequence)
- feature
- Sequentially calls a series of Runnables
- The output of one Runnable is used as the input for the next Runnable
- implementation
- Using the pipe operator '|'
- Passing a list of runnables to RunnableSequence
from langchain_core.runnables import RunnableLambda
# A RunnableSequence constructed using the `|` operator
sequence = RunnableLambda(lambda x: x + 1) | RunnableLambda(lambda x: x * 2)
sequence.invoke(1) # output 4
sequence.batch([1, 2, 3]) # output [4, 6, 8]
2.1.1.2. Parallel(RunnableParallel)
- feature
- Parallel Runnables have the same input
- Parallel Runnables each have their own output
- implementation
- Using a dict
- Passing a dict to RunnableParallel
from langchain_core.runnables import RunnableLambda
# A sequence that contains a RunnableParallel constructed using a dict literal
sequence = RunnableLambda(lambda x: x + 1) | {
'mul_2': RunnableLambda(lambda x: x * 2),
'mul_5': RunnableLambda(lambda x: x * 5)
}
sequence.invoke(1) # output {'mul_2': 4, 'mul_5': 10}
2.2. Runnable
2.2.1. What
Runnable is a unit of work that can be invoked, batched, streamed, transformed and composed, meaning it can be assembled into a Chain.
2.2.2. Function
- sync and async support
- batch support
- streaming support
2.2.3. Key Methods
- invoke/ainvoke:an input => an output
- batch/abatch:multiple input => multiple output
- stream/astream:an input => streams output
- astream_log:an input => streams output and selected intermediate results
2.3. Subclasses of Runnable
- Chain
- AgentExecutor
- BasePromptTemplate
- BaseLanguageModel
- BaseLLM
- BaseChatModel
- BaseOutputParser
- BaseRetriever
- BaseTool
2.4. Input and output types for subclasses of Runnable
2.4.1. Chain
- invoke
- input:Dict[str, Any]
- output:Dict[str, Any]
- __call__
- input:Union[Dict[str, Any], Any]
- output:Dict[str, Any]
- run
- input:Any
- output:Any
2.4.2. BasePromptTemplate
- invoke
- input:Dict
- output:PromptValue
2.4.3. BaseLLM
- invoke
- input:Union[PromptValue, str, Sequence[BaseMessage]]
- output:str
2.4.4. BaseChatModel
- invoke
- input:Union[PromptValue, str, Sequence[BaseMessage]]
- output:BaseMessage
2.4.5. BaseOutputParser
- invoke
- input:Union[str, BaseMessage]
- output:T
2.4.6. BaseRetriever
- invoke
- input:str
- output:List[Document]
2.4.7. BaseTool
- invoke
- input:Union[str, Dict]
- output:Any
3. Chain
3.1. What
- Abstract base class
- Usage:Construct sequences of calls
- Feature
- Stateful: add Memory to any Chain to give it state
- Observable: pass Callbacks to a Chain to execute additional functionality, like logging, outside the main sequence of component calls
- Composable: the Chain API is flexible enough that it is easy to combine Chains with other components, including other Chains
3.2. Attributes of Chain
- Memory:At the start, memory loads variables and passes them along in the chain. At the end, it saves any returned variables(inputs and outputs).
- Callbacks:Callback handlers are called throughout the lifecycle of a call to a chain, starting with on_chain_start, ending with on_chain_end or on_chain_error.
3.3. The Source Code Interpretation of Chain
Source Code Entrance:Chain.invoke method
Source code Location:in langchain.chains.base.py
def invoke(
self,
input: Dict[str, Any],
config: Optional[RunnableConfig] = None,
**kwargs: Any,
) -> Dict[str, Any]:
config = ensure_config(config)
callbacks = config.get("callbacks")
tags = config.get("tags")
metadata = config.get("metadata")
run_name = config.get("run_name")
include_run_info = kwargs.get("include_run_info", False)
return_only_outputs = kwargs.get("return_only_outputs", False)
inputs = self.prep_inputs(input) # Validating and preparing the input for the chain, including adding memory to input
callback_manager = CallbackManager.configure(
callbacks,
self.callbacks,
self.verbose,
tags,
self.tags,
metadata,
self.metadata,
)
new_arg_supported = inspect.signature(self._call).parameters.get("run_manager") # Checking for support for run_manager, that is, to see if the _call method has the relevant parameters
run_manager = callback_manager.on_chain_start(
dumpd(self),
inputs,
name=run_name,
)
try:
outputs = (
self._call(inputs, run_manager=run_manager) # Implementation by subclass of Chain
if new_arg_supported
else self._call(inputs)
)
except BaseException as e:
run_manager.on_chain_error(e)
raise e
run_manager.on_chain_end(outputs)
final_outputs: Dict[str, Any] = self.prep_outputs( # Validating and preparing the output of the chain and saving the information of this run into memory
inputs, outputs, return_only_outputs
)
if include_run_info:
final_outputs[RUN_KEY] = RunInfo(run_id=run_manager.run_id)
return final_outputs
source code logic of invoke method:
- prep_inputs: validating and preparing the input for the chain, including adding memory to input
- memory.load_memory_variables: related to memory
- callback.on_chain_start: related to callback
- self._call: Abstract method, implemented by subclasses of Chain
- callback.on_chain_error: related to callback
- callback.on_chain_end: related to callback
- prep_outputs: Validating and preparing the output of the chain and saving the information of this run into memory
- memory.save_context: related to memory,saving inputs and outputs into memory
4. AgentExecutor
4.1. What
- Subclass of Chain
- Intelligent agents that can use tools, have memory, and make dynamic decisions
- AgentExecutor = Agent + Memory + Tools
- Carrying out multiple rounds of iterative execution in response to user questions, ultimately returning results
4.2. Attribute of AgentExecutor
- Memory:Inherits from the parent class Chain
- Callbacks:Inherits from the parent class Chain
- Agent:Deciding the behavior of each step in iterative execution
- Tools:Tools that can be used
- Max Iterations
- Max Execution Time
4.3. The Source Code Interpretation of AgentExecutor
Step1:Chain.invoke
AgentExecutor does not override the invoke method and will call the parent class's invoke method.
Step2:AgentExecutor._call(Iterator, executing iteratively in a loop)
def _call(
self,
inputs: Dict[str, str],
run_manager: Optional[CallbackManagerForChainRun] = None,
) -> Dict[str, Any]:
"""Run text through and get agent response."""
# Construct a mapping of tool name to tool for easy lookup
name_to_tool_map = {tool.name: tool for tool in self.tools}
# We construct a mapping from each tool to a color, used for logging.
color_mapping = get_color_mapping(
[tool.name for tool in self.tools], excluded_colors=["green", "red"]
)
intermediate_steps: List[Tuple[AgentAction, str]] = []
# Let's start tracking the number of iterations and time elapsed
iterations = 0
time_elapsed = 0.0
start_time = time.time()
# We now enter the agent loop (until it returns something).
while self._should_continue(iterations, time_elapsed):
next_step_output = self._take_next_step(
name_to_tool_map,
color_mapping,
inputs,
intermediate_steps,
run_manager=run_manager,
)
if isinstance(next_step_output, AgentFinish):
return self._return(
next_step_output, intermediate_steps, run_manager=run_manager
)
intermediate_steps.extend(next_step_output)
if len(next_step_output) == 1:
next_step_action = next_step_output[0]
# See if tool should return directly
tool_return = self._get_tool_return(next_step_action)
if tool_return is not None:
return self._return(
tool_return, intermediate_steps, run_manager=run_manager
)
iterations += 1
time_elapsed = time.time() - start_time
output = self.agent.return_stopped_response(
self.early_stopping_method, intermediate_steps, **inputs
)
return self._return(output, intermediate_steps, run_manager=run_manager)
source code logic of _call method:
- while _should_continue:iterator
- _take_next_step:iterator method
Step3:Iteration Stop Condition:
- Reach Max Iterations:iterations>=self.max_iterations
- Reach Max Execution Time:time_elapsed>=self.max_execution_time
- Receive AgentFinish:isinstance(next_step_output, AgentFinish)
Step4:_take_next_step(iterator method)
def _take_next_step(
self,
name_to_tool_map: Dict[str, BaseTool],
color_mapping: Dict[str, str],
inputs: Dict[str, str],
intermediate_steps: List[Tuple[AgentAction, str]],
run_manager: Optional[CallbackManagerForChainRun] = None,
) -> Union[AgentFinish, List[Tuple[AgentAction, str]]]:
return self._consume_next_step(
[
a
for a in self._iter_next_step(
name_to_tool_map,
color_mapping,
inputs,
intermediate_steps,
run_manager,
)
]
)
source code logic of _take_next_step method:
- _iter_next_step
- agent.plan
- tool.run
- _consume_next_step
Step5:_iter_next_step
source code logic of _iter_next_step method:
- Call an LLM to generate a plan (the plan is either AgentFinish or AgentAction).
- If it's AgentFinish, return and end the process.
- If it's AgentAction, call the corresponding tool.
- Generate an observation using the corresponding tool.
- Generate and return AgentStep(action=agent_action, observation=observation), and then end.
Step6:_consume_next_step
def _consume_next_step(
self, values: NextStepOutput
) -> Union[AgentFinish, List[Tuple[AgentAction, str]]]:
if isinstance(values[-1], AgentFinish):
assert len(values) == 1
return values[-1]
else:
return [
(a.action, a.observation) for a in values if isinstance(a, AgentStep)
]
source code logic of _consume_next_step method:
- If it's AgentFinish, return directly.
- If it's AgentStep, then convert to List[Tuple[AgentAction, str]] and return.
4.4. Summary of the AgentExecutor Source Code
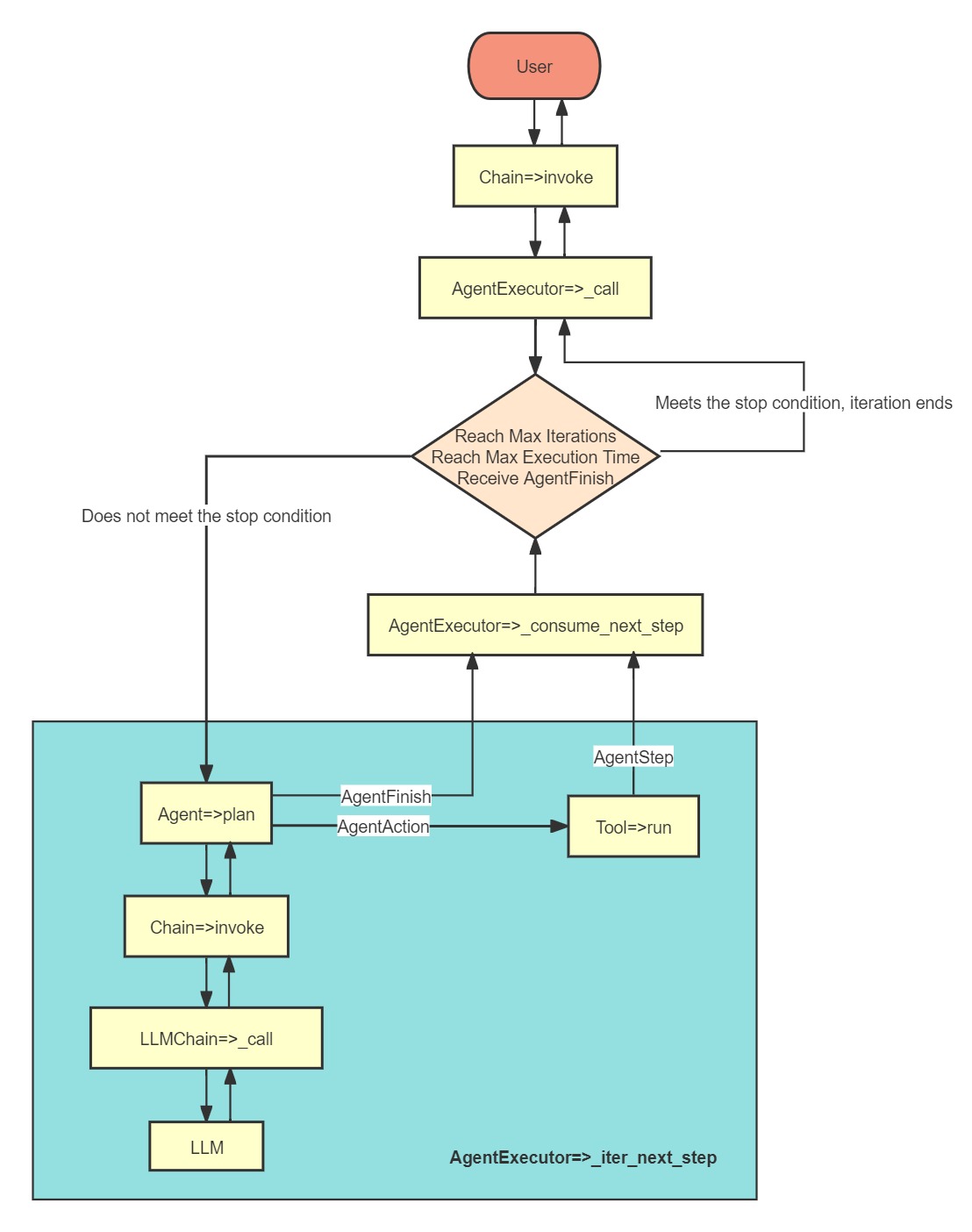